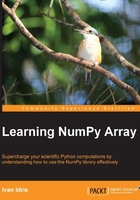
Preface
Congratulations on purchasing Learning NumPy Array! This was a smart investment, which is guaranteed to save you a lot of time Googling and searching through (online) documentation. You will learn all the essential things needed to become a confident NumPy user. NumPy started originally as part of SciPy and then was singled out as a fundamental library, which other open source Python APIs build on. As such, it is a crucial part of the common Python stack used for numerical and data analysis.
NumPy code is much cleaner than "straight" Python code that tries to accomplish the same task. There are fewer loops required, because operations work directly on arrays and matrices. The many conveniences and mathematical functions make life easier as well. The underlying algorithms have stood the test of time and have been designed with high performance in mind.
NumPy's arrays are stored more efficiently than in an equivalent data structure in base Python, such as in a list of lists. Array IO is significantly faster too. The performance improvement scales with the number of elements of an array. For large arrays, it really pays off to use NumPy. Files as large as several terabytes can be memory-mapped to arrays leading to optimal reading and writing of data. The drawback of NumPy arrays is that they are more specialized than plain lists. Outside of the context of numerical computations, NumPy arrays are less useful.
Large portions of NumPy are written in C. That makes NumPy faster than pure Python code. Finally, since NumPy is open source, you get all of the related advantages. The price is the lowest possible—free as in beer. You don't have to worry about licenses every time somebody joins your team or you need an upgrade of the software. The source code is available to everyone. This of course is beneficial to the code quality.