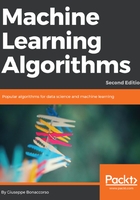
Linear models for regression
Let's consider a dataset of real-value vectors drawn from a data generating process pdata:
Each input vector is associated with a real value yi:
A linear model is based on the assumption that it's possible to approximate the output values through a regression process based on this rule:
In other words, the strong assumption is that our dataset and all other unknown points lie in the volume defined by a hyperplane and random normal noise that depends on the single point. In many cases, the covariance matrix is Σ = σ2Im (that is, homoscedastic noise); hence, the noise has the same impact on all the features. Whenever this doesn't happen (that is, when the noise is heteroscedastic), it's not possible to simplify the expression of Σ. It's helpful to understand that this situation is more common than expected, and it means that the uncertainty is higher for some features and the model can fail to explain them with enough accuracy. In general, the maximum error is proportional to both the training quality and the adaptability of the original dataset, which is proportional to the variance of the random noise. In the following graph, there's an example of two possible scenarios:

A linear regression approach is based on flat structures (lines, planes, hyperplanes); therefore, it's not able to adapt to datasets with high dispersion. One of the most common problems arises when the dataset is clearly non-linear and other models have to be considered (such as polynomial regression, neural networks, or kernel support vector machines). In this chapter, we are going to analyze different situations, showing how to measure the performance of an algorithm and how to make the most appropriate decision to solve specific problems.